Faculty Spotlight: James Pirruccello, MD
Using Deep Learning to Prevent Cardiovascular Disease
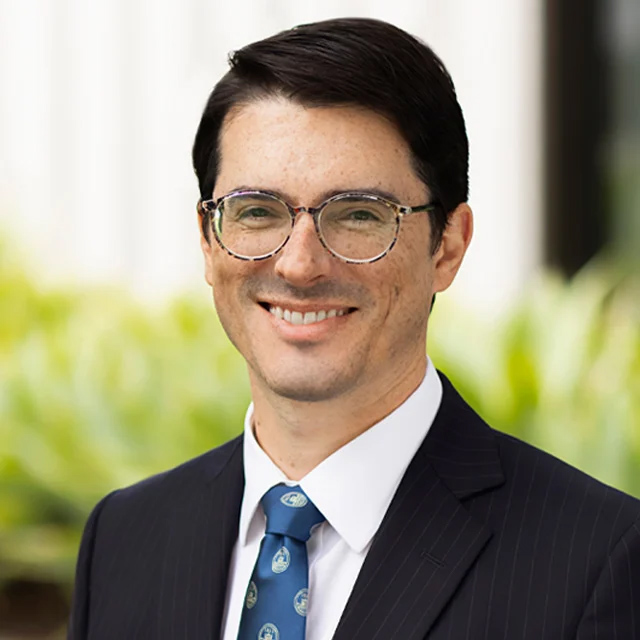
Photo credit: Andrea Rowe
James Pirruccello, MD, loved playing video games when he was growing up, and figured out how to modify what different characters said. “It gave me a taste for how you can teach devices to do anything,” he said. “That type of tinkering and figuring out how the machine worked got me started with programming.” By his early teens, he was building websites and coding.
He was also interested in medicine because it provided a way to be involved with the community, and to conduct research that could help many people. “During medical school, I needed to find a way to formally apply programming to medical questions,” said Dr. Pirruccello. “Medicine demands a lot of time, and so does programming. I didn’t want to stop either, so the best solution was figuring out how they could work together. That’s really when I got into research.”
Dr. Pirruccello uses computational methods, including deep learning – a type of machine learning model with many layers that learns from large amounts of data to make predictions. “I use these methods to derive phenotypes that are relevant to cardiovascular disease, and study the genetic basis for those traits in humans, with a focus on common genetic variation,” he said. “My end goal is to help advance the prevention of cardiovascular disease.”
Improving accuracy of health predictions could not only help doctors better diagnose and treat disease, but could also aid patients in making informed decisions. “There’s a difference between your doctor saying, ‘It would be good to lower your blood pressure. You should take this pill,’ versus ‘You’re at very high risk for developing heart failure. We think we can meaningfully reduce that risk by treating you with this pill,’” said Dr. Pirruccello. “We’re not at that point yet for all cardiovascular diseases, but I would like to help develop useful estimators of risk to identify [at-risk] patients before they develop symptoms, and support these kinds of conversations.”
Identifying Risk of Aortic Disease
He is inspired by the tremendous progress in prevention of coronary disease in recent decades. Because so many people are affected, doctors and scientists have extensively studied coronary disease and developed many effective treatments, such as statins and PCSK9 inhibitors. There are also newer technologies in the pipeline, such as gene editing to reduce cholesterol. “That’s a model that would be very useful to bring into other aspects of cardiovascular disease,” said Dr. Pirruccello. “Aortopathy is one of the conditions that seems most ripe for prevention.”
Aortopathy may cause the body’s largest blood vessel, the aorta, to tear – causing what is called an aortic dissection – or to burst. Both events are life-threatening and require immediate care. Unfortunately, aortic disease often develops silently, without causing symptoms that would allow doctors to intervene earlier with medication or surgery.
However, there are often physical changes to the structure of the aorta that can serve as early warning signs. “The aortic diameter is the best understood risk factor for aortic dissection, along with blood pressure,” said Dr. Pirruccello. Other researchers have found that the larger the diameter of the ascending aorta – the first segment of the aorta which is attached to the heart – the higher the risk of aortic dissection or rupture. However, without some sort of mechanism to identify high-risk individuals, hospitals would need to conduct imaging studies on hundreds of asymptomatic patients to identify just one with an aneurysmal ascending aorta – an unwieldy approach akin to looking for a needle in a haystack.
Dr. Pirruccello and his colleagues are using deep learning and other tools to find more practical ways to identify patients at risk of aortic disease, using existing clinical information. Their efforts have been aided by two big developments: recent advancements in machine learning, and the establishment of the UK Biobank. Created in 2006, it enrolled about 500,000 volunteers in the United Kingdom, who completed questionnaires and contributed clinical and genetic data. A subset also contributed imaging data. This rich repository is available to approved researchers all over the world.
“The UK Biobank is the only resource that has assembled large sets of both genetic and imaging data that is accessible to researchers who are not part of their group,” said Dr. Pirruccello. “They are a unique resource in multiple ways, including that they grant permission to researchers in academia or industry to use the data directly.” Rather than submitting a research question and having the UK Biobank team query the data, investigators who successfully submit an application and honor the terms of use can access the data themselves.
Dr. Pirruccello noted the care that its founder, physician Rory Collins, FRS, FMedSci, and his colleagues put into calculating the UK Biobank’s cohort size. “They thought very hard, did some math, and asked, ‘If people want to study these diseases or that imaging phenotype, how big does the sample have to be?’” he said. “That was a key insight…. Because they enrolled healthy volunteers from the general population, they needed to have a lot of people. If you are studying a disease that only affects 0.1% of the population and you only survey 1,000 people, maybe only one participant would have a measurement that fits your criteria. Anchored by that deliberate approach to science, the UK Biobank enables people like me to have a research career.”
Performing Skilled Tasks at Scale
With support from the Sarnoff Cardiovascular Research Foundation, Dr. Pirruccello and his team measured the diameter of the aorta in imaging studies from more than 30,000 UK Biobank participants. “Machine learning lets you do things at large scale that would otherwise require a huge amount of human time,” he said. “These are skilled tasks, and only cardiologists and radiologists spend their time looking at images of cardiac structure. Training [computer] models to do this is the only way to get the scale of data you need, especially from a research perspective.”
To start the machine learning process, Dr. Pirruccello creates a pre-training set of images to “teach” the computer to recognize the outline of the aorta. Because there was no off-the-shelf software tailored for this use, Dr. Pirruccello wrote his own. “That’s where it’s helpful to have a programming background,” he said. He sits in a dark room, viewing cross-section images of aortas projected onto a large screen and using an iPad to manually trace the outline of the aorta’s border. Like a medieval scribe painstakingly illuminating a manuscript, he goes pixel by pixel, taking exquisite care to mark the wall of the aorta as precisely as possible. “Whatever mistakes I make will become what the machine perceives as the correct thing,” he said. “Computer models will have errors, but I prefer the error to be because the task is difficult, not because I cut corners on entering data.”
For a relatively simple structure like aortic diameter, he spends two or three minutes tracing each image, and needed to complete about 100 images to pre-train the computer. “The aorta is probably as simple as it gets, because it’s a bright white circle on a black background,” said Dr. Pirruccello. For a more complex structure like the left ventricle, he may spend five to 10 minutes per image, and needs to trace 500 to 1,000 images for the pre-training set.
After providing the computer with these hand-annotated images, the computer then continues to “learn” by examining thousands of other images from the UK Biobank data, identifying and measuring aortic diameter for each. “This kind of approach is something that the computer vision community has been doing for decades,” said Dr. Pirruccello. “It’s nothing special for them, but for me as a doctor, it’s like, ‘Oh, this is so cool!’ It never gets old. I’m always surprised how easily a machine can be trained to do this.”
Once they were able to teach the computer to automatically measure aortic diameter with high accuracy, Dr. Pirruccello and his colleagues then tried to figure out how to predict which people were more likely to have enlarged aortas without having to image them. They developed the AORTA Score, a predictive model based on a handful of variables that are already collected in clinic visits. These included age, sex, body mass index, heart rate, blood pressure, and whether or not participants had diabetes, high blood pressure or high cholesterol. They found their model explained up to almost one-third of variance in aortic diameter, outperforming previous models and pointing the way towards improved methods for identifying patients at high risk of aortic disease in the general population.
Identifying Genetic Contributors
Genetics plays an important role in aortic disease as well. However, it’s complicated. “A key point of human genetics, especially common variant genetics, is that it’s rare for a single variant to define whether or not you’ll get a disease,” said Dr. Pirruccello. “That’s a simplified model that doesn’t really hold up for the majority of people with common diseases.” Instead, he has also used deep learning to better understand what is known as polygenic risk – identifying common variants which individually may have very weak effects on likelihood of developing disease, but collectively may have a detectible impact. These included genes that were not associated with aortic diameter, but with other features such as relative stiffness or elasticity of the aorta, a trait known as distensibility.
Dr. Pirruccello has employed deep learning in similar ways to learn more about other genotype-phenotype relationships involving other anatomical structures affected by cardiovascular disease, including the right heart, the left ventricle, and the aortic valve. With support from the John S. LaDue Memorial Fellowship, he also discovered more about titin truncated variants, the most commonly identified variants associated with dilated cardiomyopathy in adults. “Most adults found to have a titin truncating variant will not develop heart failure or heart muscle dysfunction,” he said. “The question is why. Who gets sick, and who doesn’t? We’ve demonstrated that the polygenic background has an impact on heart function even among carriers of titin truncating variants.”
While the UK Biobank is an incredible resource, Dr. Pirruccello notes the importance of also collaborating with and studying more diverse populations, such as those in San Francisco. “There are insights to be gained from the community we live in,” he said.
“Biology is universal, so if you develop a drug target, it should work across humans, because as one species we are all very similar in terms of how our bodies work,” said Dr. Pirruccello. “But for risk prediction, which is important when you’re trying to make decisions before people get sick, it’s not good if you’re only able to successfully do that for people with European ancestry.” While the U.S. has two national biobanks – All of Us and the Million Veterans Program – he would ideally also like to work with people in San Francisco to gain a deeper understanding of health and disease.
Joining a Community of Machine Learning Experts
Dr. Pirruccello was born in Portland, Ore., and grew up in Reno, Nev. He earned his bachelor’s degree in molecular biophysics and biochemistry from Yale University, and his medical degree from the Johns Hopkins University School of Medicine, spending two years as a Sarnoff Cardiovascular Research Fellow. He then completed his internal medicine residency and cardiology fellowship at Massachusetts General Hospital.
“Cardiology has a nice core of knowledge to build from,” said Dr. Pirruccello. “If someone comes in with chest pain, we have decent algorithms and a lot of clinical trial data that points us towards what we should do. It’s satisfying to have some roadmap for the more common problems in the field.”
He chose to join the UCSF Cardiology faculty in 2022 because of UCSF’s commitment to launch a genetic medicine research program, UCSF’s outstanding cardiology program, and a critical mass of colleagues engaged in related research. “Other cardiologists in the division, such as Geoff Tison and Rima Arnaout, have been very successful with their deep learning programs,” said Dr. Pirruccello. “There’s a lot of synergy in sharing ideas and talking about what is and isn’t working. By teaching each other and specializing a little bit, that pushes the field forward faster. I can learn from them, and maybe at some point they can learn from me.
“It’s also just more fun to be around people who do the same work as you,” said Dr. Pirruccello. “Feeling understood is so important. It’s nice to have people around where you can speak the same language and share your trials and tribulations – ‘Oh, my machine learning model isn't training very well. This is what I’ve tried.’ Having that strong core community of people who are doing machine learning was a very powerful draw.”
“Dr. Pirruccello brings a unique combination of expertise to our Division as a trained cardiologist, geneticist and expert in machine learning,” said Dr. Tison. “His expertise complements many in the Division, and I anticipate this will yield many valuable cross-disciplinary collaborations.”
“James comes with strong research interests and experience in cardiology critical care,” said Dr. Arnaout. “We are very fortunate to have him on faculty in the Division.”
Dr. Pirruccello appreciates the power of bringing together different areas of expertise. “I tell trainees that multidisciplinary science generally happens within a person rather than within a committee,” he said. “It’s very useful for one person to have deep expertise in one discipline but to dip their toes in multiple disciplines, because they can bring those ideas together. The best examples of success I’ve seen are when there is one person who is multidisciplinary and can bring experts from each group together, because they have a vision. That’s one of my goals.”
- Elizabeth Chur